Computer Vision Technology and Autonomous Learning Investment Strategies
By Michael Weinberg and Emily L. Spratt , Mov37
Published: 18 January 2019
In the last five years, developments in computer vision technology have reached a critical turning point largely on account of the successful incorporation of machine learning techniques into the analysis of digital images. Uses of this AI-driven technology have become ubiquitous, aiding everything from the smart searches online advertising companies offer consumers to the empowerment of self-driving vehicles. While computer vision science has begun to seamlessly influence a wide swath of industries, its potential for use in the financial sector has yet to be optimized. In this article, we consider the burgeoning role that computer vision technology, both with and without the latest AI techniques, is already serving in financial services and situate this trend within the larger movement toward computer automation in investment practices. As artificial intelligence is increasingly utilized to help navigate the plethora of data we now regularly harness to inform better business practices, we aim to bring attention to this change in the traditional methodologies employed to analyze investment information. This method we have termed Autonomous Learning Investment Strategies, or ALIS, and the use of computer vision technology is one increasingly significant tool that we can utilize in our approach to money management.
Now that information of every variety is digitally available to companies on an unprecedented scale at ever-lowering storage costs, one of the most significant problems businesses today face is effective data management and interpretation. As the sheer quantity of information requiring oversight has grown well beyond the bounds of human reach alone, data analytics has been transformed to include the now indispensable fields of computer science and statistics, which fall under the umbrella category of data science. Propelling this change in approach to information analysis has been the successful application of one AI technique in particular, deep learning, to complexly analyze structured and/or unstructured data through the use of training models that teach the machine how to complete tasks of varying levels of difficulty by way of learned examples. The application of this technique for vision technology is already having a significant influence on the interpretation of digital images and videos, and we anticipate that this will catalyze interest in the use of images to harvest information pertinent to the investment sector.
To date, the exponential growth in data, the breakthroughs in data science, the manifold applications of machine learning in business, and the record low costs of data storage and processing are reshaping the investment landscape. In the following five examples we examine current applications of computer vision technology in light of these convening factors to underscore its burgeoning market potential.
Oil: How full are the tanks? Only a few decades ago, it was not uncommon for large portfolio management companies to employ people in the Middle East to monitor and quantify the level of oil in the tanks, and to count the oil tankers and track their movement. This would then be reported back by telephone and/or fax machine. Although this was a slow process for obtaining information, it was all based on public information and anyone could have hired people to do the same thing, though few had the resources for this type of information gathering.
Fast-forwarding to our online world of digital images that record so many of our actions, we have the ability to see instantly the evidence for oil production through satellite imagery. Today, anyone can go to a tanker-tracking site and monitor daily crude oil shipments, supplies, and storage levels. Based on the satellite imagery, one can calculate the ratio of the length of the shadow inside a given tank versus the length of the shadow outside of the tank to determine how full the tank is, as exemplified in the accompanying images. With computers and satellites making these calculations, the images can be near-real time. This is an excellent example of how basic computer vision technology has replaced and disrupted the prior human role that was required for monitoring crude oil globally. ALIS managers can therefore use this computer imagery to help inform trades in crude oil and related securities.
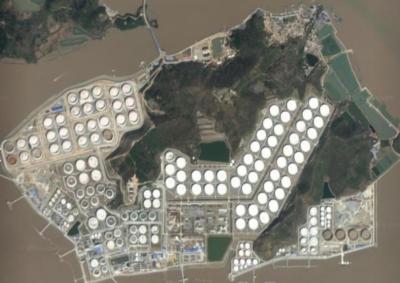
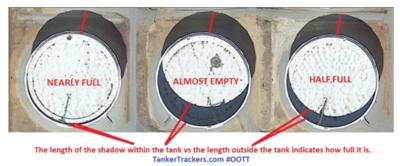
Retail: Will a company meet, beat, or miss expectations? In the past, it was often the case that portfolio managers would hire outside firms to count cars in parking lots to gather insight on the retail market. They would do this in concert with a team of people around the country. By contrast, today many of those teams have been disintermediated by the information that we derive from satellite imagery and camera surveillance of those parking lots and stores. In the image below, the large rectangular grey area represented is a big box retailer. The turquoise circles represent shoppers’ (or potential shoppers’) vehicles.
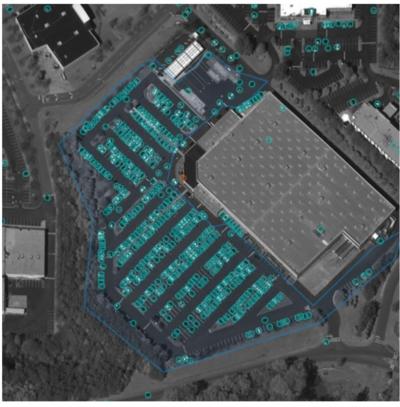
Again, depending on the periodicity of the satellite photography, one can get this information updated on a nearly constant basis. With enough historic data, ALIS managers may use this data to determine whether store traffic—and sales, which are likely a derivative of that information—are likely to meet, beat, or miss their market expectations, and invest (or divest) in the retailers’ securities accordingly. As general imaging improves, especially in regard to granularity and the frequency of image outputs, computer vision technology will allow for assessment of not only the cars in parking lots, but also the people entering stores. Consequentially, more precise forecasts of business, revenues, profitability, and security prices will be enabled.
Weather: Can we use it to forecast commodity prices? While weather forecasting is still far from infallible, improvements are also possible through the analysis of highly dimensional datasets with low signal-to-noise ratios. For example, ALIS managers may monitor the weather at what were previously inconceivable levels of granularity and frequency. While public forecasts may have certain biases that are intentional, such as the underreporting of the probability of rain occurring (for the reason of not depressing the populace), ALIS managers have the ability to directly make weather predictions given the availability of weather data from the two key providers of this information. Furthermore, they may combine these forecasts with other satellite imagery and computer vision data to gain more precise answers to pertinent investment questions relating to environmental conditions.
Let us consider the example of one’s approach to trading energy futures. If one uses satellite imagery and determines that there is a massive snow cover on the solar panels in a certain region that may diminish the output of solar energy, one can by extension predict higher prices for fossil fuels. In addition, one may use satellite imagery to discern equipment failures on wind-driven turbines, which may similarly have an adverse impact on the supply of that power source and the resultant impact on the pricing of alternative power sources. Consequently, these ALIS managers may be able to predict commodity yields and prices ranging from agriculture to energy more accurately than previously possible or based on public weather forecasts. This record level of data and imagery is unprecedented and a boon to ALIS managers’ data arsenals, which could potentially generate outsized returns and alpha.
Sports Images: Can we use them in betting? ALIS funds in Europe are using machine learning techniques to analyze digital imagery of games to generate returns and alpha in sports betting. These ALIS managers are not expressing personal preferences; instead, they are systematically using machine learning, data, data science, and record low processing and storage costs to try to gain an edge to predict which teams are likely to win and lose and where the odds are mispriced, just as an investor might do when investing in companies and commodities.
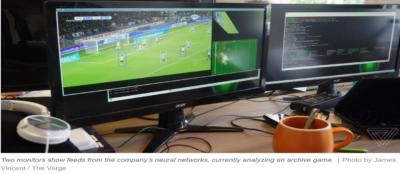
The upper image shows two monitors from a company’s neural network analyzing an archived soccer game. Other computer vision programs examine the 3-D images of bodies in videos to enhance content analysis and even to predict the percentage likelihood of a goal by estimating the poses of the figures relative to each other.
Heart Rates: Predicting prevarication from video analysis Through supervised learning and off-the-shelf web cameras, it is possible to estimate the heart rates of persons from videos focused on faces. Imagine a public company executive on a webcast, at a conference, or on television. Examining the forecasted data of the executive’s heart rate based on video of that individual, it is possible to hypothesize whether the person was prevaricating. This might have implications on the veracity of the executive’s statements and result in buy, sell, or hold recommendations on the company. With further diminished cloud-driven processing and storage costs, an ALIS manager could not only analyze the current imagery but also compare it to prior imagery of the same person for greater accuracy. Recurring patterns of dishonesty may also be discerned by tracking videos of certain persons.
Overall, digital technology, the increased resolution of digital photographs, and the declining cost of camera hardware and software will in turn further the ability to use computer vision technology, which is also being propelled by the advances in machine learning, as an ALIS tool. Further declines in the storage of photographs and videos will similarly facilitate the increased usage of photos and videos. Especially considering the data consumptive nature of videos to date, we do not believe that this medium for the dissemination of information has been adequately considered, let alone exploited, for ALIS, and that this will quickly change with better data storage solutions.
What Is the Future of Computer Vision Technology as an ALIS Tool?
We would like to suggest that computer vision science is one of the most promising fields of study regarding the analysis of our increasingly digital visual world. While processing and storage costs of image-related data need to fall even further to meet their fullest potential for ALIS, we see much potential in the use-value of digital image analysis in finance, and predict that this area of data analytics will grow significantly. As our society becomes as fluent in image-based information exchanges as in text-based communications, the way we analyze our world of digital images has the potential to become a fast and effective way to measure our behaviors and their socio-economic impact.
For instance, by utilizing image recognition technologies empowered by deep learning techniques, the incidence of the appearance of images on social media related to particular products or company brands could be tracked over time as one useful signal, amongst others, as an indicator of market sentiment. It is important to highlight, however, that the visual landscape of our digital world is not a neutral indicator of the behavior of markets, as visual replication, biases, and outright fraud create noise in the data, which require much disambiguation. (The use of bots to amplify the dissemination and seeming influence of a given set of information is but one reason for trepidation.) We therefore predict that the most successful applications of computer vision technology in this environment will be tempered with management strategies that effectively account for intentional and unintentional skewing of a given dataset. We also would note that as with all data usage, ALIS managers must ensure that they behave responsibly and have the right to collect any given dataset and use it with due respect for information rights and digital privacy.
In conclusion, as ALIS managers harness the value provided by alternative, unstructured, nonfinancial data, including image data, the value of the effective and accurate interpretation of it is becoming more apparent. As more capital, and, consequently, research and development are invested in directing the growth of image recognition technology, its return as an investment tool will also become more apparent. Given the notable developments in computer vision technology, it is possible to navigate the investment landscape with machine-empowered eyes of the world. The better we can decipher our digital world of images, the greater transparency we will find in our analysis of the markets.