Data challenges in new high yield digital lending markets for asset managers
By Altin Kadareja; Marco Masotto; Luca Tiozzo, CARDO AI
Published: 28 November 2022
Credit markets are evolving quickly, and new asset classes are emerging every day. Asset managers are always on the lookout for the most promising opportunities, but they are not always prepared to face the challenges these new asset classes entail. Take, for instance, innovative credit models such as Buy Now Pay Later (BNPL) and Revenue-Based Financing (RBF). When it comes to data, these types of financing structures carry with them a unique and novel set of challenges.
Without the proper tools and processes in place, investments’ data is difficult to manage, monitor, and reconcile. A lack of efficient data management can result in wrong decisions, which in turn lead to higher defaults and lower performance, hampering an asset manager's ability to fully capitalise on the emerging opportunities inside the credit markets.
After a brief overview of the emerging credit models, we will examine the data challenges they present and how market actors can successfully overcome them.
Buy Now Pay Later
The general Buy Now Pay Later model involves a customer purchasing on the merchant’s website with BNPL financing. When the purchase is confirmed by the merchant - and by the BNPL provider - the customer receives its products.
From a financial point of view, every time a new product is purchased with the BNPL option, a new loan is issued by the BNPL provider and - in case of securitisation - sold to an SPV.
Clients benefit from a free payment split over time (max 3/4 installments), and asset managers have access to portfolio diversification, and attractive returns (generally paid by the merchant).
Revenue-based financing
In revenue-based financing, a company funds its financing needs with a loan to be repaid from a share of its future revenues. For the borrower, this becomes a very flexible and scalable instrument as there will be no ‘large’ installment at the end of the month, but only a fixed percentage of sales applied on a daily basis.
On the other hand, the lender receives back the capital and interest much faster and in a more predictable way. The relationship between the two is key to this business model. The financing line becomes true growth support for the borrower instead of a ‘burden’ that would come from a classical loan.
Inventory-based financing
Inventory-based financing involves using inventory as collateral to obtain an advance, loan, or line of credit to purchase products/inventory or fund liquidity short-term cash flow problems. In the last few years, this financing model has extended to retail and seasonal businesses, including product categories ranging from clothing to food and beverages.
This model represents an attractive form of financing for businesses and lenders. Since inventory is used as collateral, it is easier for a business to obtain a loan compared to other types of financing, while from a lender’s perspective, there is less risk associated with the investment.
eCommerce financing
Marketplaces such as Amazon wait 14 - 60 days before making the payment to the retailer after a transaction is completed. Marketplaces retain consumer payments for a certain amount of time to facilitate returns and refunds, but retailers struggle in managing their operations with such long cash cycles.
eCommerce financing enables merchants to get their cash at the end of each sales day. The faster cash cycle supports the merchants’ growth while reducing working capital. For a lender, the attractiveness of eCommerce financing derives from the visibility of the historical cash-flows and revenue data.
Device as a service
The device/product as a service business involves the rental of a device/tech product/car/ etc., to the final customer. Compared to BNPL, this financing model provides a greater payment guarantee (if the customer stops paying, the lender will take back the product), decreasing the risk associated with the investment.
This form of financing allows customers to rent devices in the way that best suits their needs. There is no upfront capital expense which significantly lowers the barrier to entry to try new devices or vehicles.
Data challenges in new digital lending markets for asset managers
Distinctive business models
As the credit models described above are relatively new to the market, there is still no standard investment management structure in place. This becomes particularly evident for BNPL products: while some providers charge a fee to the merchants on generated sales, others employ a subscription-only or a mixed model with a fixed subscription and fee on sales. In some cases, BNPL providers give the option to extend the installment schedules further, applying interest on the outstanding amount.
It often happens that asset managers invest in several BNPL providers. This adds even more complexity as each provider, depending on its geography, has specific accounting and reporting policies, data, and business models, and so on, leading asset managers to lose track of realised performance.
Continuous modifications to repayment schedules
Asset managers must carefully plan and monitor their investments on an ongoing basis. Predicting the expected tenor and return of a single exposure or a portfolio is critical, however, the lending models discussed above do not make it an easy task to accomplish.
The above-mentioned asset classes are usually managed at borrower level, allowing consolidation of exposure generated via multiple purchases. In many cases, extensions or modifications of amortisation plans are granted, generating further changes to the payment schedule.
Asset managers must review their expected cash flows and returns over and over again usually using .csv files
Tracking all these changes in maturities and returns is rather difficult considering exposure is usually granular and spread over thousands of lines. In addition, when business models are based on fixed subscription fees (as in the case of many BNPL products), there is no direct connection between the borrowed amount and the paid fees: the subscription fee would still be the same and will still be charged regardless of whether or not the credit line is used.
Furthermore, every single BNPL provider has its own specific way of reporting data, increasing the complexity of monitoring activities to understand how investments perform. All of the above combined make it extremely challenging to monitor delinquencies, defaults, delays, and, more broadly, investment performance.
Volatility
With revenue-based finance, returns can be extremely volatile. Given the product structure, with no fixed maturity and repayments that correlate to company revenues, planning in advance is not straightforward, as revenues can be uncertain and fluctuate over time depending on the borrower’s monthly performance.
From a lender (or asset manager) perspective, revenue based finance is somehow more similar to equity than to debt, as its value can be considered as a revenue multiplicator. Therefore, in order to plan efficiently, every quarter, asset managers need to revise and adjust estimations, the lent amount, and expected returns. Managing cash expectations efficiently is quite complex and, as a consequence, there could be a high cash drag effect and lower returns for the clients.
eCommerce financing presents similar issues, especially when it comes to product returns (refunds), which represent a big risk for the lender. Once a sale is recorded and the financing is extended to the merchant, a refund request from the customer causes a credit drop, decreasing the borrowing base while increasing the risk and the expected tenor (which in turn reduces returns).
Full visibility on investments
Proper management of Inventory-based financing requires asset managers to constantly track what is happening to the underlying assets. Devaluations, weight reductions, and other factors could impact the collateral’s value. Being able to retrieve reliable data that enables efficient and time-effective monitoring might require dedicated solutions.
In order to have full visibility of all these changes, asset managers need to rely on automated tools that can predict in real-time returns, automatically adjusting statistics and generating alerts based on the past data and characteristics of the scenario under evaluation.
Need for custom-made reporting
Traditional tools such as spreadsheets imply very manual and tedious reporting processes, with a high level of operational risk. Emerging credit models require personalised adjustments each time, which increases the difficulty of accommodating reporting needs of external parties quickly and accurately.
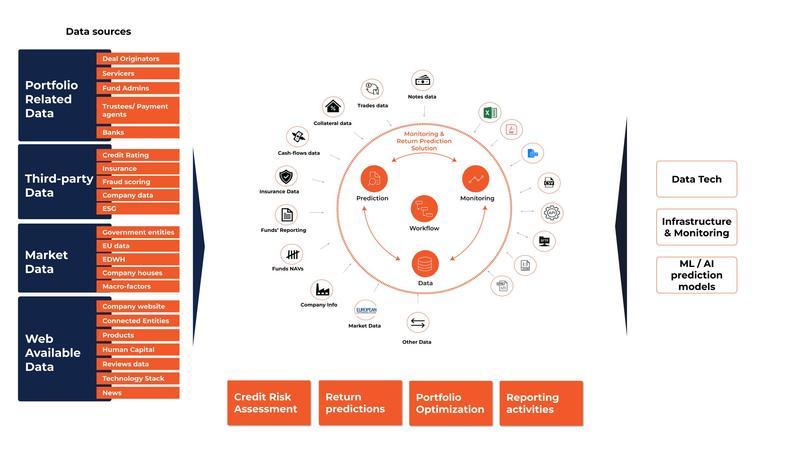
Streamlined data management process with AI and ML-based models
Conclusion
“With great opportunities come great challenges” and technology has the potential to solve them all by providing the right tool to correctly manage data and bring transparency and speed to asset managers.
However, using software with basic functions, in the case of new lending models, is simply not enough. The market needs dedicated AI-based models that are built based on each specific product structure. For instance, BNPL products require delay prediction models, propensity to pay back models, and revenue limit estimations that are automatically calculated with every new subscription and updated after each event (purchase or repayment). Standard calculations updated on a yearly basis are not sufficiently detailed.
Moving at the same speed as the market is critical to stay ahead of competitors. When evaluating whether to enter the emerging credit markets, asset managers should take into account all the data challenges they entail and think in advance about a dedicated solution to streamline operations and analytics.